Exploring AI's Impact on Modern Financial Applications
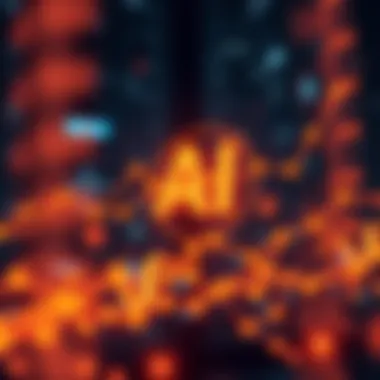
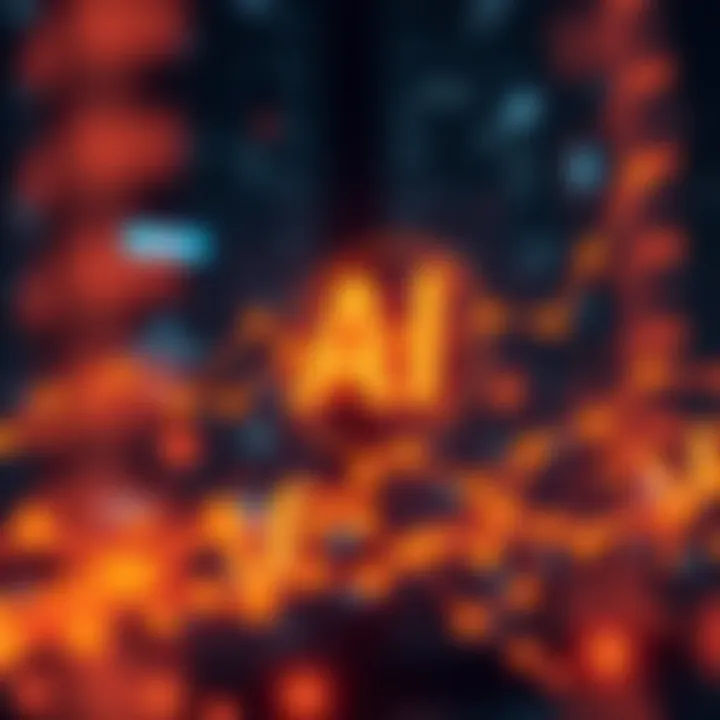
Intro
In the expansive terrain of finance, artificial intelligence (AI) stands as a major player, ready to shift paradigms and redefine the way investors interact with the market. Modern financial applications powered by AI have become synonymous with efficiency and precision, enabling stakeholders to navigate an intricate web of data, automate decision-making processes, and even enhance customer experiences. This expansive influence is apparent across various sectors, each leveraging AI in distinct but synergistic manners.
As the landscape evolves, key terminologies begin to surface, and understanding these terms is vital for capitalizing on AI’s capabilities. From algorithmic trading to customer service chatbots, each element plays a role that is not only significant but transformative. The aim of this delving narrative is to break down these components for both novice and experienced investors, ensuring that clearer insights are achieved in this increasingly automated world.
As we embark on this exploration of AI’s role in finance, we’ll investigate relevant applications, the implications of these tools, and a glimpse into the upcoming trends that are poised to reshape critical financial interactions. Buckle up, because there’s quite a journey ahead, full of insights that might just change how you perceive finance and investment decisions.
Investment Dictionaries
Terminology Breakdown
Understanding the terminology related to AI in finance can feel a bit like diving into deep waters without a life jacket. With terms ranging from machine learning to predictive analytics, it’s crucial to have a strong grasp on what these words mean.
- Algorithmic Trading: This refers to the use of AI algorithms to execute trades at high speeds with minimal human intervention.
- Roboadvisors: Automated platforms offering financial advice or portfolio management services, often utilizing algorithms to tailor responses based on client data.
- Predictive Modeling: The analysis of historical data to predict future market trends, helping investors make informed decisions.
- Natural Language Processing (NLP): A branch of AI that allows machines to understand and interpret human language, often used in chatbots for customer service.
Practical Examples
To illustrate how AI jargon translates into real-life applications, consider the following scenarios:
- A hedge fund utilizes algorithmic trading to make split-second decisions, capitalizing on minute fluctuations in stock prices. This technology can process vast amounts of data and execute trades far faster than a human ever could.
- A retail investor might turn to a roboadvisor after a recommendation from a friend. By inputting information about their financial goals, the roboadvisor creates a personalized investment strategy without requiring a face-to-face meeting.
- An insurance company employs predictive modeling to not only assess risk better but also set competitive pricing for their policies based on projected claims.
As we move forward, it becomes clear that understanding this terminology not only enhances comprehension but also enables informed decision-making. The path through these complexities might seem daunting at first, but it becomes less tangled with knowledge at your fingertips.
“The only thing worse than being a novice is remaining one through ignorance.”
In the ever-changing world of finance, opinions may vary on what constitutes a wise investment strategy. The following sections will further scrutinize various AI applications and their implications, establishing a clearer view of the terrain ahead.
Prolusion to Artificial Intelligence in Finance
Artificial Intelligence is becoming a cornerstone in the ever-evolving world of finance. Its role is not just supplementary; it is transforming how financial institutions operate, how investors make decisions, and how products are developed. The integration of AI brings with it a host of advantages, from efficiency gains to enhanced accuracy in predictions. This section aims to shed light on what AI entails within the context of finance and why it is essential for both professionals and consumers alike.
Defining Artificial Intelligence
Artificial intelligence, often referred to as AI, can be simplified to mean the simulation of human intelligence processes by machines, especially computer systems. These processes include learning—acquiring information and the rules for using it; reasoning—using rules to reach approximate or definite conclusions; and self-correction.
In finance, AI encompasses several subsets of technology, including machine learning, natural language processing, and expert systems. For instance, when a machine analyzes vast datasets to forecast market trends or assess risk, it embodies AI in practical action. Such activities can save professionals a considerable amount of time and reduce the likelihood of human error.
Historical Context of AI in Finance
Looking back, the roots of using AI in finance can be traced to the late 20th century when advanced algorithms began emerging. Initially, the concept of algorithmic trading emerged as a way for speedy transactions to outperform human traders. As technology advanced, so did the complexity of these algorithms, allowing for high-frequency trading that executes orders at lightning speed.
By the 2000s, companies began pouring significant investment into AI research and development, exploring its potential for risk assessment and customer interaction. The 2008 financial crisis acted as a catalyst, revealing the limitations of traditional forecasting methods. Consequently, many firms sought out AI solutions that could process and analyze data at a scale far beyond human capability. This shift was not merely reactive; it marked the beginning of proactive approaches in financial planning and risk management.
"The trajectory we are witnessing with AI in finance is akin to the Industrial Revolution; it's changing the very fabric of how we approach financial services."
In summary, the journey of AI in finance has been a testament to innovation and adaptation. The technological landscape that AI occupies today is vast, with implications reaching into every corner of the financial world. As our discussion progresses, the intricacies and advantages of this technology will further illuminate its vital role in contemporary finance.
AI in Investment Strategies
Artificial Intelligence is fundamentally transforming investment strategies in ways that were once the stuff of science fiction. For investors, understanding how AI integrates into these strategies is crucial. It’s not just about the flashy headlines of billion-dollar deals; rather, it’s about how AI powers the mechanisms behind these investments with unmatched precision and efficiency. This section takes a closer look at the ins and outs of various applications of AI in investments and its significance in the modern financial landscape.
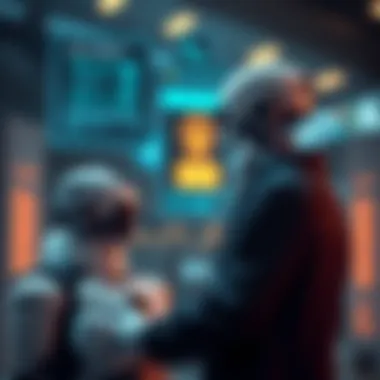
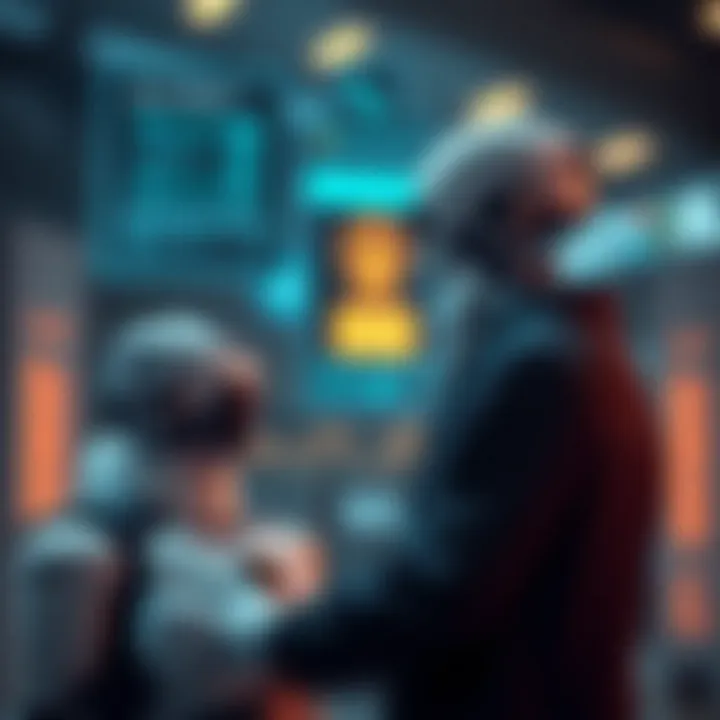
Algorithmic Trading Overview
Algorithmic trading is at the forefront of financial technology, serving as a quick and efficient way to execute trades based on complex algorithms. Imagine a speed chess player, one who can consider countless moves in mere seconds. AI operates on this principle, analyzing vast datasets to determine the best times to buy or sell.
- Speed is the name of the game. AI systems can analyze market conditions and adjust strategies in real-time, outperforming human traders without fatigue or emotion.
- Data-Driven Decisions are another significant aspect. Unlike the traditional methods that often rely on intuition or past experience, algorithmic trading places heavy emphasis on statistical models and data trends. Investors are armed with insights that can lead to better buy-sell decisions.
These advantages create a ripple effect, influencing prices, improving liquidity, and sometimes even leading to flash crashes. Therefore, while algorithmic trading brings opportunities, it also requires careful consideration of its risks.
Data Analysis and Predictive Modeling
Data is the oil of the 21st century, and predictive modeling using AI has become a vital tool for investors. Picture a clairvoyant who doesn't just predict rain but helps people prepare for it. Predictive models utilize machine learning algorithms to profile market trends and forecast future outcomes based on historical data.
Key elements include:
- Historical Data Patterns: AI sorts through mountains of past data to identify trends that may not be apparent to the human eye.
- Sentiment Analysis: With access to news feeds and social media, AI assesses the mood around certain assets. This is akin to gauging public opinion in a political race—knowing how the winds are blowing can guide investment decisions.
This blend of advanced analytics and predictive capabilities allows investors and fund managers to create strategies that adapt to an ever-changing market landscape. However, reliance on algorithms also introduces a layer of complexity regarding transparency and potential algorithm biases.
Risk Management through AI
Understanding risks is like dancing on a tightrope; one small misstep can lead to catastrophic results. AI plays an instrumental role in risk management through the development of sophisticated risk assessment models. By analyzing a wide array of factors—from market volatility to geopolitical events—AI can provide a clearer picture of potential risks.
Consider these aspects:
- Scenario Analysis: AI can run simulations on different investment scenarios, revealing how various factors may impact returns.
- Real-time Monitoring: Continuous evaluation of market conditions means that AI can alert investors when risks surge unexpectedly. This is somewhat akin to having a fire alarm that notifies you the moment there's smoke.
In summary, AI enhances risk management by providing granular insights that empower investors to make informed decisions. The integration of AI into investment strategies not only optimizes engagements but also requires a solid understanding of the nuances and challenges it presents. As technology continues to evolve, the onus is more on investors to adapt their strategies and remain aware of the limitations and ethical implications that accompany AI advancements.
It’s essential to strike a balance between the efficiency brought by AI and the instinctive human touch that many traditional investors still cherish.
By delving into these three subsections, we see how AI is not just a tool, but a game changer that demands both rational caution and innovative thinking from investors navigating today’s dynamic financial landscapes.
Customer Interaction and Support
In the financial sector, customer interaction and support are crucial components that can make or break a client’s experience. With the rise of artificial intelligence, institutions can provide more tailored, efficient, and responsive services, ensuring client satisfaction and loyalty. The integration of AI in this area not only enhances user engagement but also streamlines processes that traditionally required significant human resources. This shift comes with its own set of advantages and challenges, making it an essential topic in understanding AI's role in finance.
Chatbots and Virtual Assistants
Chatbots and virtual assistants have become ubiquitous in customer support across various sectors, and finance is no exception. These AI-driven tools are designed to handle a range of client queries, from simple account inquiries to more complex transactions, all while providing instantaneous responses.
One of the primary advantages of using chatbots is the 24/7 availability they offer. No longer are clients left waiting for business hours to resolve their issues. This constant access can significantly improve customer satisfaction, as clients appreciate quick resolutions, which can directly impact their trust in financial institutions.
Moreover, the use of natural language processing enables these AI tools to communicate in a more human-like manner. This creates a sense of familiarity and comfort for users that might otherwise feel hesitant to reach out for assistance. However, despite the technology's prowess, there remains a need for human oversight to manage more nuanced queries effectively.
Personalization in Client Services
In today's world, personalization is king, especially in the financial domain. Clients increasingly expect services tailored specifically to their financial needs and preferences. AI excels here by analyzing vast amounts of data to identify trends and preferences unique to each user.
For instance, banks may utilize algorithms to offer personalized product recommendations based on transaction histories and individual financial behavior. Imagine a situation where a bank sends you tailored investment options that align with your interests and risk appetite. This not only saves time but also enhances the relevance of the services offered.
Furthermore, through advanced data analytics, institutions can foresee customer needs even before they arise, enabling proactive engagement. Such strategies are paramount as they set firms apart in an increasingly competitive landscape.
AI-Driven Financial Advising
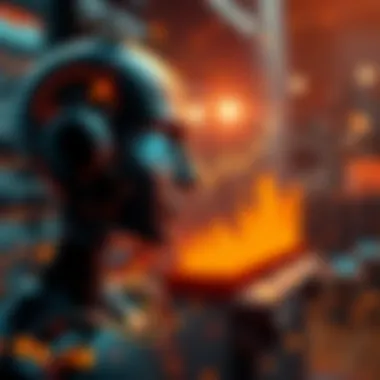
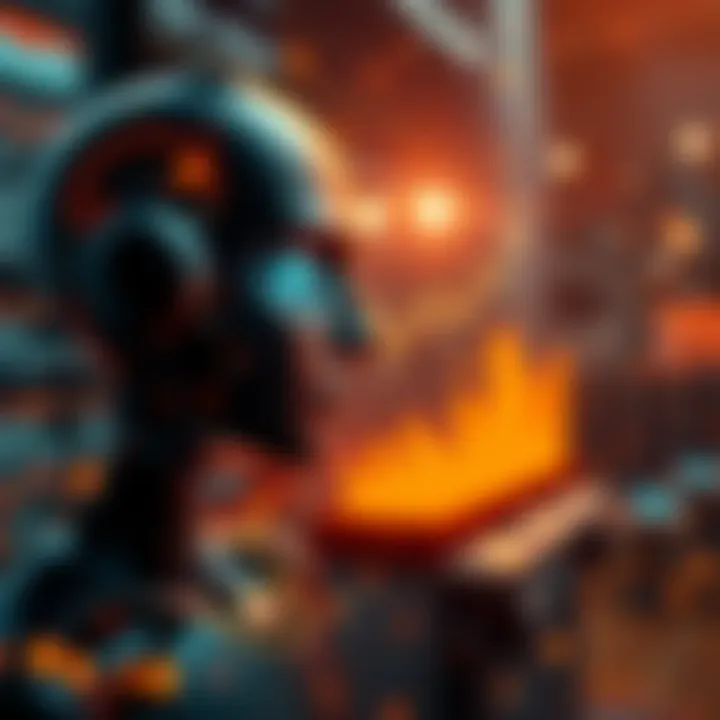
AI-driven financial advising is paving the way for a new era in investment strategies and wealth management. Unlike traditional advisors, these AI systems can process and analyze massive datasets at lightning speed, providing insights that would be impossible for human advisors alone.
For example, tools like Betterment and Wealthfront utilize algorithms that help clients create and manage investment portfolios tailored to their objectives. This opens up financial advising to a broader audience, including younger generations unfamiliar with complex investment jargon.
However, while AI advisors can provide valuable insights, there are certain limitations to consider. The risk of over-reliance on AI can lead to significant challenges, especially when market conditions shift unexpectedly. The human touch in understanding subconscious biases and emotions related to finance remains unmatched, calling for a balanced approach in using AI-driven advice alongside traditional financial counseling.
"AI in finance doesn’t replace human advisors; rather, it enhances their capabilities by diving deep into data analytics, freeing them up to focus on personal relationships with clients."
In summary, the role of AI in customer interaction and support within the financial sector is transformative. It empowers institutions to deliver personalized, efficient services while balancing technology with humanity for optimal results.
AI's Impact on Financial Products
In an era where money management is becoming more complex, AI plays a pivotal role in reshaping financial products. These innovations improve the precision and speed of transactions, enhance user experiences, and unlock untapped markets by providing tailored solutions to diverse consumer needs. The relevance of AI in the context of financial products cannot be overstated, as it not only streamlines operations but also propels institutions towards a more data-driven future.
Automated Investment Platforms
Automated investment platforms, often referred to as robo-advisors, have gained significant traction over the past decade. They employ algorithms to manage portfolios by utilizing data analytics which traditionally required human insight. This technology lowers the entry barrier for investing, making it accessible to a wider audience, including those who might have felt intimidated by conventional investments.
From Vanguard to Wealthfront, these platforms offer diverse services ranging from managing retirement accounts to providing tailored investment advice. An investor can start with as little as a few hundred dollars, and the algorithms continuously re-balance portfolios according to market conditions, investment objectives, and risk tolerance.
Benefits can include:
- Cost Efficiency: Automated systems reduce management fees significantly compared to traditional advisory services.
- Diverse Portfolio Creation: Algorithms can quickly explore vast datasets to recommend a broad range of assets, including stocks, bonds, and ETFs.
- Real-time Adjustments: These platforms continually analyze market data, allowing for quicker adaptations to changing financial climates.
However, some challenges remain. Investors must place considerable trust in automated systems, which can sometimes lack the human touch in terms of understanding emotional or psychological factors affecting investment decisions. Additionally, there are concerns about algorithmic bias leading to less than optimal investment choices.
Credit Scoring and Lending Decisions
In the lending arena, AI has revolutionized how credit scores are calculated and how lending decisions are made. Traditional credit scoring methods often fail to provide a complete picture of a person’s creditworthiness. They generally rely on historical data which can disadvantage younger individuals or those new to credit.
AI algorithms analyze a broader range of data points, including spending habits, account balances, and even social media activity, to construct a more holistic view of credit risk. Companies like Upstart are employing this technology to assess potential borrowers, thus reducing the fraction of individuals deemed unqualified due to conventional scoring limits.
Advantages include:
- Faster Decision Making: AI speeds up the process of credit approval, benefiting both lenders and borrowers.
- Access to Credit: By considering unconventional metrics, more individuals can secure loans, potentially including those from underserved communities.
- Reduced Risk: A more thorough analysis of data enables lenders to mitigate risks associated with default.
Yet, there are ethical implications tied to these advancements. There is always a risk of perpetuating bias if the data fed into these systems isn't thoroughly vetted. Transparency becomes a pressing issue, as consumers may not fully understand how their data is used in the decision-making process.
"The integration of AI into financial products is reshaping the landscape, leading to both unprecedented opportunities and significant ethical challenges."
In summary, the impact of AI on financial products is profound and multifaceted. Automated investment platforms democratize investment for the masses, while enhanced credit scoring mechanisms provide more equitable lending opportunities. As these technologies continue to evolve, navigating the accompanying ethical concerns will be vital for fostering trust and ensuring that the benefits of AI are equitably distributed.
Ethical Considerations in AI Development
In the fast-paced world of finance, the deployment of artificial intelligence raises not just questions about efficiency and profit but also serious ethical concerns. These considerations weigh heavily as they influence both the financial sectors' reputation and its relationship with users. It's essential to tackle ethical issues head-on to ensure that AI applications are not only effective but also trustworthy.
The significance of addressing ethical considerations in AI development can be boiled down to several critical elements:
- Trustworthiness: In finance, trust is paramount. If consumers feel that AI systems operate with bias or lack transparency, it could erode confidence in financial institutions.
- Fairness: As AI takes a more prominent role in decision-making, it is crucial to ensure that these systems support equitable outcomes and do not reinforce existing disparities.
- Accountability: When AI systems make decisions, who is responsible for those outcomes? Clarity in accountability ensures that all parties remain answerable, making for a more robust regulatory environment.
Bias in AI Algorithms
Bias in AI algorithms poses a significant challenge. Algorithms are only as good as the data fed into them. If historical data is flawed or skewed, AI can perpetuate these biases, leading to unfair treatment of certain groups. For example, banks could inadvertently use credit scoring models that disadvantage minorities due to biased historical lending practices.
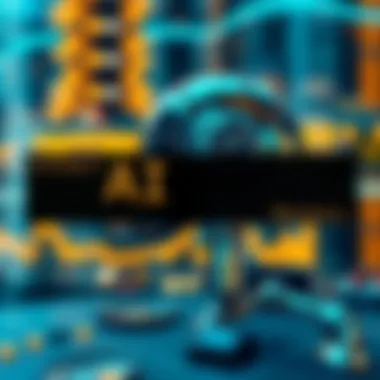
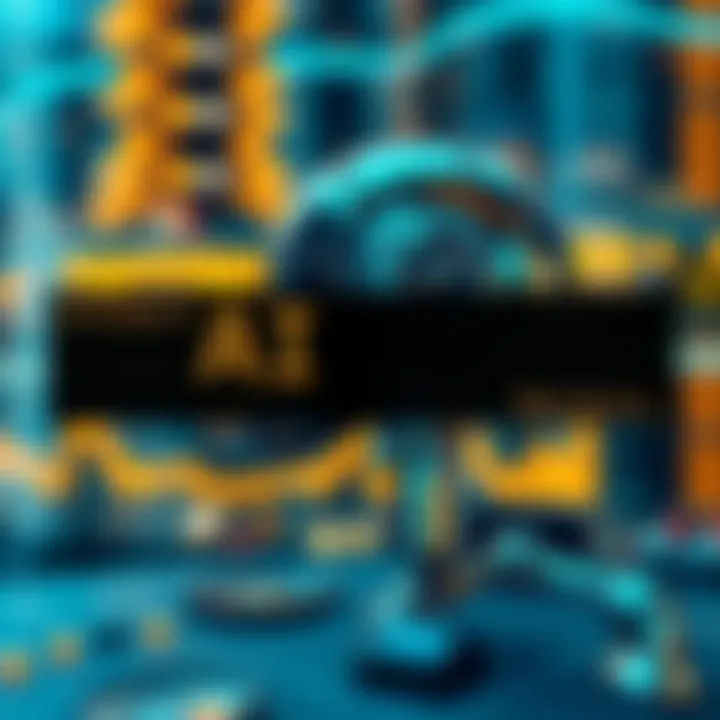
Recognizing and mitigating bias begins with understanding the datasets used to train these AI systems. The financial sector must be proactive in reviewing and refining these data sources to eradicate any unjust influence. This includes diversified testing, continuous monitoring of outcomes, and adjustment processes for algorithms that appear to favor certain demographics over others.
Bias in AI isn't just a technical issue; it's a societal one that carries significant implications for equity in finance.
Transparency and Accountability
Transparency in AI operations fosters trust, allowing users to understand how decisions are made. The more clients comprehend the processes behind AI-generated outcomes, the more likely they are to buy into them. Financial institutions must provide clear explanations about how AI models work. This includes disclosing the algorithms used and the rationale behind specific decisions.
Additionally, accountability must be clearly defined. Designating accountability means ensuring that there’s a human element ready to take responsibility for decisions made by AI. This human oversight is crucial for navigating complex ethical landscapes and for holding institutions accountable in instances of errors or injustices.
Key strategies to enhance transparency and accountability include:
- Regular audits of AI algorithms to assess their performance and outcomes.
- Open communication with clients about the role of AI in their financial products and services.
- Establishing clear channels for clients to question decisions made by AI systems and receive prompt, reasoned responses.
At the end of the day, building ethical AI is about creating systems that prioritize human values alongside technological advancement. Balancing innovation with responsibility is not just a goal; it's an ongoing commitment vital for the future of finance.
Future Trends in AI and Finance
The fusion of artificial intelligence and finance is undergoing rapid evolution. As financial markets get increasingly digitalized, there’s a growing reliance on advanced technologies to navigate complexities. One of the key trends is the integration of AI, which promises to redefine traditional finance frameworks. Investors, financial planners, and those in the academic sphere are all keenly observing these developments.
Beyond just efficiency, the implications of these innovations touch on security, decision-making, and regulatory adherence. Understanding these trends equips professionals to not just keep up, but potentially leverage advancements for competitive advantage.
Integration of Blockchain and AI
Merging blockchain technology with artificial intelligence can feel like the best of both worlds, offering immense potential for the financial sector. Here’s why this integration is pivotal:
- Transparency and Security: Blockchain provides a decentralized and immutable ledger, ensuring that transaction records are secure and verifiable. When AI is layered on top, it can analyze these transactions, detect anomalies, and improve security measures.
- Enhanced Data Insights: AI can process large volumes of data from blockchain transactions, unveiling patterns and trends that were previously hidden. This capability can shift how investment strategies are developed, reducing risk and enhancing transparency.
- Decentralized Applications: Smart contracts powered by AI can automate processes that traditionally required manual supervision, leading to increased speed and efficiency in executing contracts and agreements.
The combination of these technologies may also revolutionize areas like payments and lending. It lowers barriers, pushing traditional financial entities to rethink their current operations. The collaborative potential encourages innovation that meets the demands of a tech-savvy consumer base.
AI in Regulatory Compliance
In a landscape where regulations can feel like a never-ending jungle, AI serves as a guiding light for compliance. The sheer force of data that financial institutions handle mandates an agile and proactive compliance strategy. Here’s how AI can aid in adhering to regulations:
- Real-Time Monitoring: AI systems can conduct constant surveillance of transactions and client interactions to identify potential non-compliance issues instantly, acting before they escalate.
- Predictive Analytics: Utilizing historical data, AI models can predict potential risks associated with regulatory breaches, thus enabling organizations to mitigate issues before they arise.
- Simplification of Reporting: Generating compliance reports traditionally requires substantial human effort. AI can streamline this process, automatically compiling and analyzing data to align with regulatory standards efficiently.
"AI doesn’t just facilitate compliance; it transforms the way organizations envision and execute their regulatory strategies."
With the stakes so high in managing compliance risks, organizations aiming for efficiency must embrace AI. This way, they not only adhere to regulations but can also pivot swiftly in response to regulatory changes.
In sum, the future trends in AI and finance highlight a trajectory shaped by technological advancement and strategic integration. Stakeholders who grasp these concepts will undoubtedly be positioned to harness the benefits that come with them.
Ending
In summarizing the significant role of AI within the financial sector, it becomes clear that this technology is not merely an enhancement but a revolutionary force. AI enables financial institutions to harness vast amounts of data, leading to smarter decision-making and greater efficiency. By understanding the nuances in AI applications, stakeholders can better navigate the future of finance, which is increasingly intertwined with advanced technologies.
The Continuing Evolution of AI in Finance
AI is not static; it's evolving rapidly, reshaping the landscape of finance as it does so. Today, we witness continuous advancements ranging from machine learning algorithms that analyze market trends to natural language processing systems that improve client interaction. Moreover, as financial markets become more complex, the fusion of AI with other cutting-edge technologies like blockchain offers promising solutions that enhance security and transparency in transactions.
The journey of AI's integration into financial services is akin to a river winding its way through a valley—it continuously adapts and carves new paths. Customer experiences are becoming more personalized, efficient, and informative, thanks to AI's role in tailoring services to meet individual needs. These developments signal a future where finance is not only aligned with innovation but is also accessible to a broader audience, fostering financial literacy and empowerment.
Balancing Innovation with Responsibility
While the ability of AI to drive innovation is significant, it should be matched by a strong commitment to ethical considerations. Financial institutions must tread carefully to avoid pitfalls associated with bias in algorithms that could inadvertently affect lending decisions or investment advice. The push for greater transparency and accountability cannot be overstated.
One way to promote responsible AI deployment is through robust regulatory frameworks that prioritize consumer protection without stifling innovation. In this balancing act, open dialogues among regulators, industry players, and the public are vital. Only by prioritizing ethical standards can we ensure that the advantages of AI are realized by all segments of society, rather than being perceived as tools serving the interests of the few.
"The most valuable asset in the age of AI is not just data, but the ethical use of that data, ensuring it benefits the collective rather than undermines it."